What exactly is Big Data?
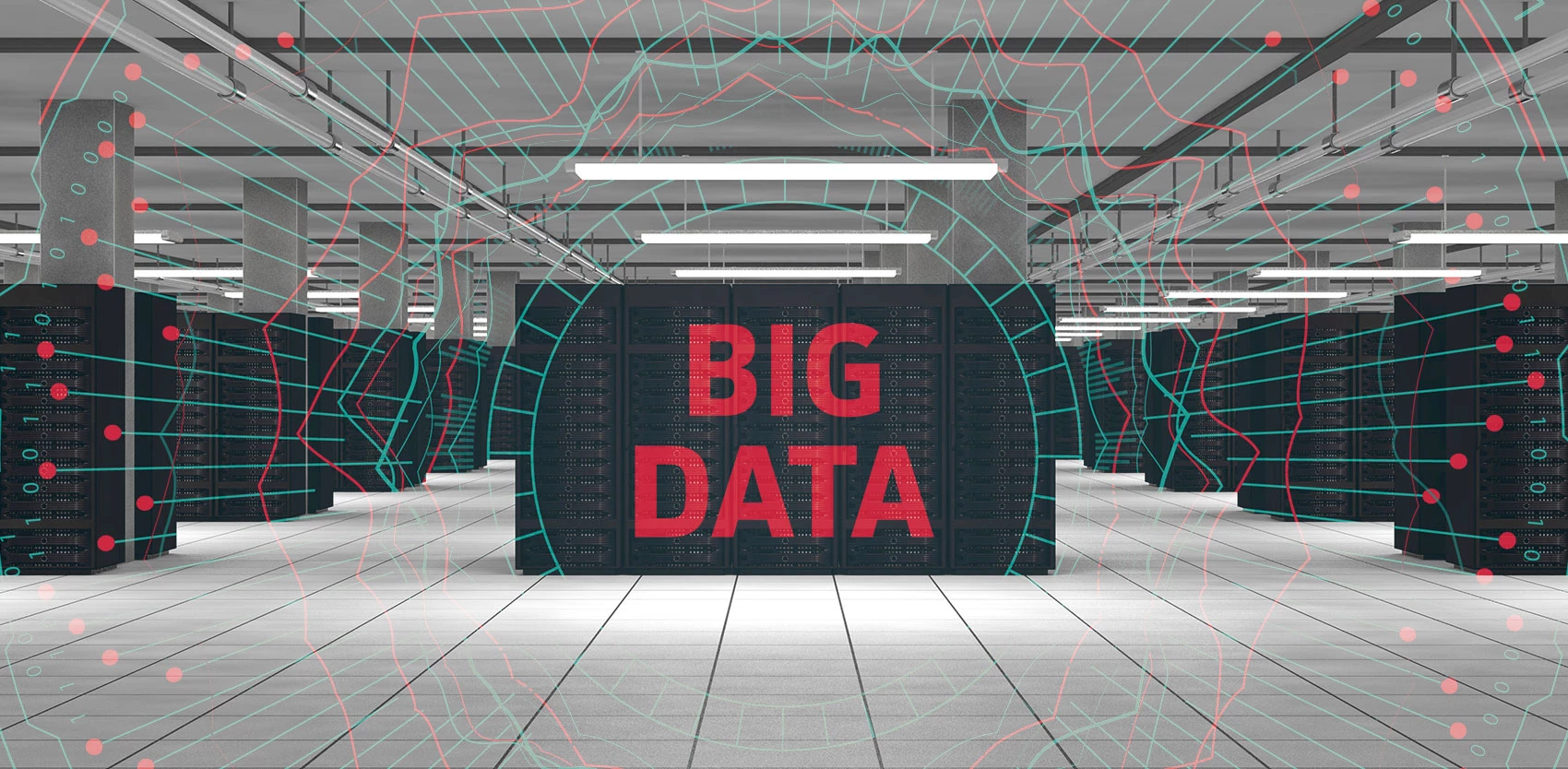
Big Data is what everyone’s talking about. Its long list of successes has us enthralled. But the reality is far more prosaic: Big Data is simply the science of managing huge (and growing) volumes of varied data and mining them to extract information valuable enough to make it cost-effective.
The concept of Big Data was born in the 1990s, when major enterprises started to acquire ever-larger information assets, but it crystallized in the early 2000s. Doug Laney, an analyst at Meta Group (bought out by Gartner in 2005), coined the generally accepted three-V definition of Big Data: high volume, velocity and variety.
Volume
Data production is exploding across the board. Digital information is being produced by an increasing number of technologies, while storage solutions are getting cheaper, faster and larger. Companies that recognize information as a potential source of value hate to throw out any of it and choose to store it for future use.
In 1998, IBM launched the Deskstar 25GP hard drive, with a 25GB capacity, the largest at the time for PCs. It cost about US$200 (US$510 in 2018 dollars). Today, a 1-terabyte drive (i.e. 1,000GB) sells for US$50. This amount of storage in 1998 would have required 40 Deskstar drives and an outlay of US$20,400. In just 20 years, the price of 1 GB has dropped from US$20.50 to US$0.05.
In the 1990s, due to its cost, companies chose the frugal approach to data storage. Today (not to mention tomorrow), cost is no longer an issue, and companies are keeping everything, regardless of whether it may ever come in handy. And thanks to the digital revolution and attendant connected objects (the Web, mobile apps, etc.), enterprises and individuals are producing ever more data. In fact, data production is growing at an exponential rate.
Velocity
Data flows are larger and faster than ever, and modern computational methods allow for real-time processing in most situations. Thanks to the Internet, to wireless local networks, to mobile phone networks and to IoT-dedicated networks, a flood of data is flowing from sensors to data storage and analysis systems. Thanks to increasingly powerful processors, this data can be swiftly analyzed and useful information extracted almost instantaneously.
For example, in the past, a monitoring system for manufacturing machinery would produce data that was locally stored and periodically transferred to a processing system on some sort of physical medium. Today, this same monitoring system is equipped with a sensor that can be directly and continuously connected to the whole world. The information is produced, stored and processed in the blink of an eye.
Variety
As data-producing devices become more varied and ubiquitous, the data they produce is also more varied, in terms of format and structure. Text, images, audio and video files, structured and unstructured data: a river of data flows in standard and non-standard formats into the ocean of Big Data.
Using Big Data
Big Data is useless without appropriate analysis and mining tools. These tools must overcome the challenge of the three Vs: they must be able to process a great volume and variety of data, very quickly. The ultimate goal is to enable companies to create value, optimize processes, and gain a competitive edge by turning reams of data into strategic information. For example, companies might want to detect early trends that would otherwise remain unnoticed, in order to act more quickly and deliberately. Or they could use data to feed predictive models to gauge the market’s reaction to a new offering before actually launching it.
Today, analysis tools are improving rapidly thanks to artificial intelligence and especially deep learning, which has the ability to cope with data variability. Faced with diverse data, AI is able to extract meaning from audio and video files, recognize images, translate texts, structure raw data, make semantic links between disparate data, make correlations and detect subtle patterns, resolve inconsistencies, etc.
Note that the main obstacle encountered in Big Data projects is data quality. If you provide bad data (erroneous, fragmented, inconsistently structured), the best artificial intelligence system will not be able to do much with it and, at worst, will produce faulty analysis.